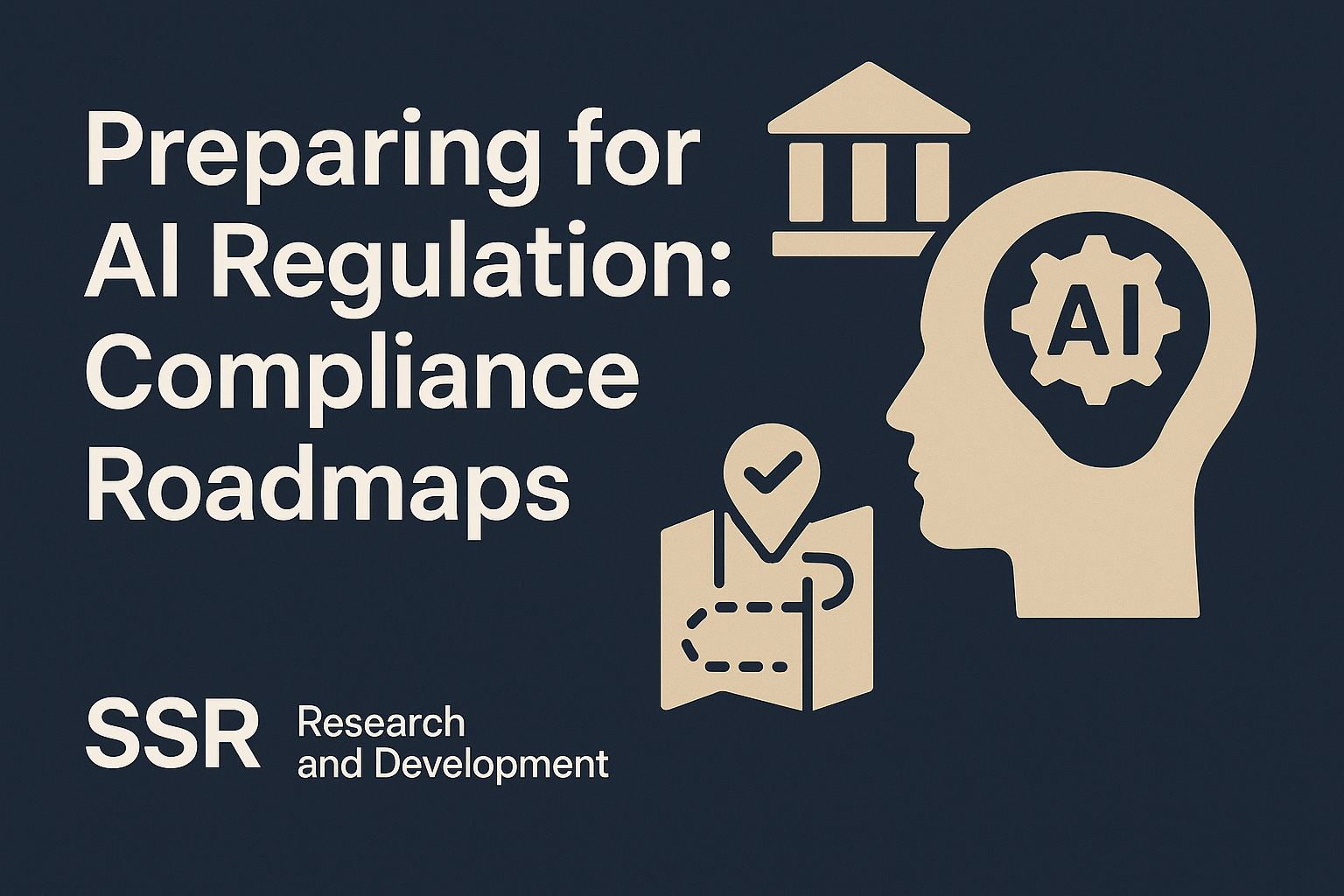
Introduction
Artificial Intelligence (AI) is transforming business operations across industries, but this rapid adoption comes with increasing regulatory scrutiny worldwide. Governments are introducing AI-specific regulations to ensure ethical and responsible AI integration, aiming to protect consumers and uphold fundamental rights. Preparing for these rules is now a strategic imperative for businesses of all sizes, not just a legal exercise. A forward-looking compliance roadmap can help organizations navigate emerging requirements while aligning with ethical AI practices. This research article outlines key global AI regulations. It offers practical guidance on building a compliance program, documenting AI systems, assessing regulatory readiness, and designing AI systems that adapt to evolving frameworks. The goal is to equip businesses with the knowledge and tools to meet current and future AI obligations responsibly and responsibly.
Overview of Major AI Regulations Globally
The EU AI Act: The European Union’s AI Act is a landmark framework setting the tone for global AI governance. It uses a risk-based categorization of AI systems into tiers (unacceptable, high, limited, and minimal risk), with obligations increasing for higher-risk uses (Kompella, 2024). Unacceptable AI systems (e.g., social scoring or subliminal manipulation) are banned outright. In contrast, high-risk AI systems (such as AI in employment, education, essential services, law enforcement, etc.) are permitted but heavily regulated (Kompella, 2024). Providers of high-risk AI must implement rigorous risk management, data governance, documentation, monitoring, and human oversight measures and ensure their systems meet standards of safety, accuracy, and robustness (Kompella, 2024). They must also undergo conformity assessments before the market launch to prove compliance. The EU AI Act imposes strict penalties for non-compliance – fines can reach the greater of €30 million or 7% of global annual revenue (Kompella, 2024). Although final technical requirements and standards under the Act are being finalized, the law is expected to fully apply by 2025–2026, after a transition period. The EU framework’s comprehensive approach and steep penalties (comparable to the GDPR in impact) have made it a global reference point for AI governance.
Emerging U.S. Regulatory Developments: In contrast to the EU, the United States, as of 2025, has no single omnibus AI law at the federal level. Instead, the U.S. relies on a patchwork of existing laws and agency guidelines while efforts are underway toward new AI legislation (White & Case LLP, 2025). Federal authorities have issued soft-law guidance – for example, the White House’s Blueprint for an AI Bill of Rights and the National Institute of Standards and Technology’s AI Risk Management Framework (AI RMF) – to encourage trustworthy AI practices. Regulatory enforcement is currently achieved through existing laws (such as anti-discrimination, consumer protection, or privacy statutes) applied to AI contexts, alongside warnings from agencies like the FTC that deceptive or biased AI outcomes can trigger liability. Meanwhile, activity has shifted to the state level: several U.S. states are advancing their AI regulations. Colorado’s new AI law (Colorado Artificial Intelligence Act), enacted in 2024, is the first comprehensive state AI statute focused on “high-risk” AI systems used in consequential decisions (Levi et al., 2024). The Colorado law mandates numerous compliance steps – including documentation, risk assessments, governance programs, and algorithmic impact assessments – for developers and users of high-risk AI (Levi et al., 2024). It also references frameworks like NIST’s AI RMF as a baseline for risk management, with an expectation that controls be scaled appropriately to the organization’s size and the system’s impact (Levi et al., 2024). As more states introduce AI bills, companies operating in the U.S. face a growing patchwork of state AI laws, underscoring the need for a robust, adaptable compliance strategy in the absence of a single federal rule (Levi et al., 2024).
Other Global Regimes: Outside the EU and the U.S., many jurisdictions are rapidly shaping their AI governance approaches. The United Kingdom has opted for a principled, sector-led strategy: rather than an AI Act, the UK published guidelines for regulators to apply existing laws to AI and plans to introduce legislation emphasizing a pro-innovation approach in 2025. China has moved quickly to implement strict AI rules: authorities issued regulations on algorithmic transparency and generative AI services, and a draft PRC AI Law (2024) outlines comprehensive requirements akin to the EU’s, with an added focus on national security and specified high-risk use categories (Babin, 2024). Some countries, such as the United Arab Emirates, enforce AI requirements in specific sectors (e.g., requiring AI ethics self-assessments in certain industries) while relying on voluntary guidelines (Babin, 2024). Others, like Japan and Singapore, have released voluntary AI governance frameworks and codes of conduct to guide the industry without binding rules (Babin, 2024). International bodies are also active – the G7 nations proposed a voluntary code of practice for AI, and the OECD’s AI principles promote global norms for trustworthy AI.
In summary, the regulatory landscape is quickly evolving worldwide: the EU has set a high bar with a comprehensive Act, the U.S. is developing a combination of guidelines and emerging laws, and many other governments are following their mix of regulations or guidance. Businesses must monitor these developments in all jurisdictions where they operate. Despite differing approaches, a common theme is emerging – accountability, transparency, risk management, and human oversight are core expectations of AI systems everywhere.
Best Practices for an AI Compliance Program
Given the fast-changing regulatory environment, organizations should establish a formal AI compliance program to ensure their use of AI stays within legal and ethical boundaries. A robust program is not one-size-fits-all – it should be scaled to a company’s size and industry – but there are key best practices that apply to any business integrating AI (Shirkhanloo, 2025; Kompella, 2024). Below are important components for creating an AI compliance program that aligns with current and evolving regulations:
- Governance and Oversight: Set up an AI governance structure with clear accountability. Define roles and responsibilities for AI oversight (e.g., an AI risk committee or officer). Senior leadership should endorse AI governance policies to signal that compliance and ethics are prioritized (Kompella, 2024). An AI governance framework establishes guiding principles (fairness, transparency, privacy, safety) and integrates them into the AI development lifecycle. As new laws emerge, a governance body that can adapt policies in real-time will help the organization stay compliant (Shirkhanloo, 2025). Cross-functional involvement is crucial – legal, compliance, IT, data science, HR, and business units should collaborate on AI policy setting and risk reviews to ensure all perspectives are covered (Shirkhanloo, 2025).
- Inventory of AI Systems: Catalog all AI systems in use or development across the organization (Kompella, 2024). For each system, document its purpose, how it works (e.g., machine learning model type), and whether the company is the “provider” of the AI (developing it) or merely a “deployer”/user of a third-party AI product. This inventory is the foundation for compliance: it allows mapping each AI system to applicable regulations based on its risk domain. For instance, identify which systems might be considered high-risk (e.g., those used in hiring or lending decisions) and, therefore, subject to specific legal requirements. Maintaining an updated AI inventory also supports transparency and accountability efforts.
- Risk Assessment and Management: Implement a process to assess the risks of each AI system, both at design time and periodically during operation. This includes evaluating the potential for bias or discrimination, privacy impacts, safety or reliability issues, and any harm resulting from unintended use. Many regulations require or encourage Algorithmic Impact Assessments (AIAs) or similar risk assessments for high-impact AI (Hilliard, 2024). As part of the compliance program, develop an AI risk management framework (Shirkhanloo, 2025). This can build on existing enterprise risk management practices but be tailored to AI specifics: for example, follow the structure of NIST’s AI RMF, which recommends functions like governing, mapping, measuring, and managing AI risks throughout the AI lifecycle. Organizations can more easily fulfill regulatory expectations by extending traditional risk controls to cover AI (e.g., bias testing as a risk control, or model performance monitoring as an internal control) (Kompella, 2024). High-risk AI systems should undergo rigorous pre-deployment testing and periodic re-evaluation to ensure that risk mitigation measures remain effective. Document all findings and mitigation steps as evidence of due diligence.
- Policies for Procurement and Third-Party AI: Incorporate compliance considerations into procuring AI products or services (Kompella, 2024). The compliance program should require vetting vendors to ensure their adherence to AI regulations if using third-party AI tools (such as cloud AI services or purchased algorithms). Include questions about how vendors address bias, transparency, and upcoming laws in RFI/RFP processes (Kompella, 2024). Contracts with AI providers should contain provisions around data usage, model updates, and compliance – for example, requiring the vendor to notify of significant changes to the AI system or to cooperate with compliance audits (Shirkhanloo, 2025). Managing third-party AI risk is increasingly important, as regulators may hold companies accountable for harms caused by the AI tools they deploy. Therefore, due diligence on suppliers (ensuring they follow frameworks like the AI Act or NIST standards) is a best practice.
- Training and Culture: Provide training on AI compliance and ethics to stakeholders at all levels (Kompella, 2024). Technical teams (data scientists, developers) should be educated on upcoming regulatory requirements – for instance, what documentation they need to produce or what bias testing is required. Non-technical teams (management, HR, legal, audit) also need awareness of AI risks and responsibilities. Regular workshops or seminars can cover topics like data quality standards, how to detect and mitigate bias, privacy protection in AI, and the organization’s specific AI policies (Kompella, 2024). Building a culture of “responsible AI” is key: employees should feel accountable for the impacts of AI systems they design or use. Encouraging ethical considerations and caution (e.g., asking, “Could this algorithm unintentionally discriminate?”) will complement formal compliance steps and help prepare the organization for stricter rules.
- Internal Auditing and Monitoring: Treat AI systems like other critical processes subject to internal controls. Establish an internal AI audit function or integrate AI into existing compliance audits (Kompella, 2024). Periodically review AI systems for compliance with policies and regulations – for example, verify that required documentation is up to date, test that bias controls are working, and confirm that only approved data is being used. Unlike one-time product audits, AI audits should be ongoing because AI models can evolve or drift over time (Shirkhanloo, 2025). Developing capabilities for continuous monitoring (such as automated alerts if an AI model’s outputs begin to deviate or show bias) can help catch issues early. An internal audit might also simulate a regulator’s perspective: checking if the AI system would pass an external assessment under laws like the EU AI Act or sector standards. Findings from these audits should feed back into improving the compliance program. In short, regular “check-ups” on AI systems ensure compliance is not a checkbox at launch but a sustained effort throughout the AI’s life.
By implementing the above practices, an AI compliance program becomes a living, adaptive system within the organization. This proactive approach positions businesses to handle new regulatory mandates with less disruption. It also aligns with ethical AI principles – many practices (transparency, fairness checks, accountability structures) meet legal requirements and foster responsible AI that stakeholders and customers can trust.
Documentation Requirements for Regulated AI Systems
Emerging AI regulations place heavy emphasis on documentation and record-keeping for AI systems. Detailed documentation serves as evidence of compliance and a mechanism for transparency into otherwise “black-box” algorithms. A robust documentation practice should cover the entire AI system lifecycle. Key documentation requirements – reflected in the EU AI Act and other proposals – include the following:
- Data Provenance and Quality: Document the origin and characteristics of datasets used in developing and operating AI systems. Regulations expect organizations to maintain information on where training and test data were obtained, how the data were collected or labeled, and any relevant preprocessing steps (ISACA, 2024). For high-risk AI, one must record the provenance of data – including the data source, any third-party data suppliers, and the methods used to ensure data accuracy and relevance (ISACA, 2024). Documentation should also address data quality issues and bias mitigation, for example, noting if certain groups are under-represented and what steps were taken to correct for potential bias. By tracking data lineage and quality, businesses can demonstrate compliance with data governance requirements in AI laws (and also with privacy laws like GDPR that intersect with AI data use).
- Model Development Lifecycle: Maintain thorough documentation of the AI system’s development process and technical specifications. This includes a description of the model architecture and algorithms, the AI system’s intended purpose and use cases, and how it interacts with hardware or other software (ISACA, 2024). All phases of the model lifecycle should be covered: design decisions, training procedures, validation results, and deployment context. For instance, companies should record the version of the model, training epochs, performance metrics achieved (accuracy, error rates, etc.), and the outcomes of any robustness or security testing (ISACA, 2024). If the model is updated or improved over time, each modification and new version should be documented along with the reason for changes. Regulators want a traceable record of “what was built and how” for AI systems. This readily available documentation is crucial for compliance audits and useful internally for debugging and model governance.
- Impact Assessment and Risk Analysis Reports: Many frameworks now call for conducting Algorithmic Impact Assessments (AIAs) or similar evaluations for high-impact AI systems (Hilliard, 2024). Any such assessment should be documented in a report. An AIA report outlines the system’s context, the potential harms or biases identified, and mitigation measures taken. Under the EU AI Act’s draft provisions and some national laws, deployers of high-risk AI may be required to perform a fundamental rights impact assessment before implementing the system. In the U.S., proposed legislation like the Algorithmic Accountability Act would mandate impact assessments for AI used in critical decisions (Hilliard, 2024). Even when not explicitly required by law, documenting an AI system’s ethical and societal impact is a best practice. It demonstrates that the organization has proactively considered privacy, fairness, and safety implications. Similarly, if bias or fairness audits are conducted (as recommended by many guidelines), the methodology and results of those audits should be recorded. By keeping a paper trail of risk assessments, companies can show regulators that they have an iterative process to identify and mitigate risks (Shirkhanloo, 2025). Notably, such documentation must be kept up to date – if an AI system is modified or its use changes, the impact assessment should be revisited and the documentation updated accordingly.
- Audit Trails and Logging: Regulations increasingly require that AI systems maintain automatic logs of their operation, especially for high-risk use cases (ISACA, 2024). For example, the EU AI Act mandates that high-risk AI systems log their activities to ensure traceability. These logs should capture when and how the system was used and key inputs/outputs. Concretely, an audit trail might include the timestamps of each decision made by the AI, the input data or query that triggered the AI’s decision, and the output or recommendation generated (ISACA, 2024). If human overseers reviewed or intervened in the AI’s decision, that should also be logged. The identity of personnel who verified results or performed tests can be recorded for accountability (ISACA, 2024). Comprehensive logging allows an organization or regulator to later audit the system’s behavior – for instance, to investigate an incident or to verify compliance. Businesses should have procedures to securely store these logs and prevent tampering. Additionally, documentation should describe the logging setup and how long records are retained. Audit trails are helpful for compliance; they can facilitate post-market monitoring of AI performance and help detect anomalies or drifts in the system over time.
In summary, regulated AI systems require extensive documentation akin to a “technical dossier.” This dossier provides transparency into the AI’s design, data, and performance and evidences the organization’s compliance efforts (ISACA, 2024). Companies should establish templates and processes to generate and maintain these documents as part of their standard AI development workflow. Good documentation practices not only satisfy regulators but also improve internal governance of AI – by making complex systems more understandable and traceable. When regulators (or auditors) come knocking, having this documentation ready will significantly simplify the demonstration of compliance.
Regulatory Readiness Assessments for AI Systems
With new AI regulations on the horizon, organizations should regularly conduct regulatory readiness assessments of their AI systems. A regulatory readiness assessment is essentially an internal check (or a third-party evaluation) to gauge how well an AI system and the surrounding processes meet current and expected legal requirements. This proactive measure can identify gaps before regulators do, allowing time to address issues and strengthen compliance controls.
Baseline Compliance Gap Analysis: The first step is to compare existing AI systems against applicable laws and guidelines requirements. For example, a company operating in Europe might review a high-risk AI system against each obligation in the EU AI Act: Is there a risk management system in place? Is technical documentation complete? Are human oversight measures implemented? If the system falls short on any requirement, those gaps are noted. Similarly, a U.S. company might assess an AI tool against the NIST AI RMF or the principles from the AI Bill of Rights, as these frameworks often anticipate regulatory expectations (Shirkhanloo, 2025). This gap analysis now helps teams understand what changes or controls will be needed when new rules come into force. Many organizations are extending their existing compliance checklists (for privacy, cybersecurity, etc.) to include AI-specific checkpoints – for instance, verifying that an AI that makes hiring recommendations can be audited for bias, as would be required under emerging hiring AI laws.
Simulation of Conformity Assessments: In jurisdictions like the EU, formal conformity assessments will be required for specific AI systems (those deemed high-risk) before they can be deployed or sold. These assessments, often performed by accredited third parties or through internal compliance review, evaluate whether the system adheres to all regulatory criteria (ISACA, 2024). Organizations can prepare by doing a trial run – essentially a mock audit of the AI system. This involves reviewing all the documentation (as described in the prior section), testing the system’s outputs for compliance (e.g., does it meet accuracy thresholds set by the law?), and ensuring the required oversight and safety mechanisms are in place. Suppose the company finds deficiencies during this simulated audit. In that case, it can take corrective action (e.g., improve the model or add a needed feature) before the actual conformity assessment or regulator review. In the U.S. context, while there is no single conformity process, companies might simulate an FTC investigation or an external audit by looking at their AI through fairness and transparency expectations. The key is to adopt the perspective of regulators or certifiers: be stringent and assume they will ask for evidence on each compliance point.
Internal Audits and Continuous Evaluation: A readiness assessment should not be a one-off project—it needs to be embedded into ongoing compliance monitoring. As part of the AI compliance program, organizations must conduct periodic internal AI audits (Kompella, 2024). These audits review technical aspects (model behavior, data handling) and procedural aspects (whether documentation is kept up, incident response processes for AI issues exist, etc.). Internal audit teams or independent reviewers can use checklists derived from regulations. For instance, after the EU AI Act is fully enforced, an internal audit one year later might check if the company’s high-risk AI systems have been registered in the EU database as required and whether any new risks have emerged in operation. If regulations change or new laws pass (which is likely in the coming years), the readiness assessment criteria should be updated accordingly. This is where having an adaptive governance model is valuable: legal and compliance teams should continuously track legislative developments and update the organization’s internal standards to match (Shirkhanloo, 2025). By doing so, when a new rule takes effect, the company’s AI systems are already close to compliant by design.
Regulatory Sandboxes and External Reviews: In some cases, regulators offer “sandboxes” or pilot programs where companies can have their AI systems evaluated in a controlled environment. Participating in these can be a good way to assess readiness with regulatory feedback. Additionally, companies may hire external experts (consultants or law firms) to perform an independent compliance assessment. An external review can provide an objective look and often mirrors what a regulator or certified auditor would do. The result is a report highlighting any compliance weaknesses and recommendations. Incorporating such external assessments periodically (for example, annually for the most critical AI systems) can add credibility to the company’s compliance efforts and ensure nothing is overlooked.
In conducting regulatory readiness assessments, organizations should also consider multi-jurisdictional compliance. An AI system deployed globally might need to meet the strictest requirements among all applicable regimes. A readiness check might reveal, for example, that a system meets current U.S. expectations but not all EU provisions – prompting the company to upgrade the system (perhaps by adding an explanation interface or more rigorous documentation) to be universally compliant. Forward-thinking companies choose to comply with the “highest common denominator” of regulations, turning compliance into a competitive advantage by meeting or exceeding what laws require across markets.
Ultimately, being prepared is far better than being caught off-guard. Regulatory readiness assessments give management assurance that the company can pass an official audit or inquiry. They also signal to employees that compliance is an active, ongoing concern. This preparedness can save companies from frantic last-minute fixes, enforcement penalties, or reputational damage in the fast-evolving AI regulatory landscape. By identifying and addressing compliance gaps early, businesses can confidently innovate in AI, knowing they are building on a solid, lawful foundation.
Designing Adaptable AI Systems for Changing Regulatory Frameworks
Given the dynamic nature of AI technology and the laws governing it, businesses should strive to design adaptable AI systems – systems and processes flexible enough to meet evolving regulatory requirements. Rather than viewing compliance as a one-time checklist, organizations are urged to adopt a “compliance by design” mindset for AI development, similar to the established concept of privacy by design. This means baking regulatory considerations and ethical principles into the AI system from the outset and throughout its lifecycle so that adjustments can be made with minimal disruption as rules change.
Modular and Transparent System Architecture: One approach to adaptability is building AI solutions modularly. For instance, if you separate the core machine learning model from the user-facing interface and the logging subsystem, updating one component without overhauling everything becomes more manageable. A modular logging component can be upgraded to capture that info if a new law requires storing additional data about AI decisions. Likewise, designing for transparency and explainability from the start will pay off when regulations demand it. Many AI models, incredibly complex deep learning ones, are opaque. To future-proof against likely mandates for explainability, companies can incorporate explainable AI techniques (such as model-agnostic explanation tools or inherently interpretable models) during development (Shirkhanloo, 2025). By implementing these features early, the AI system will be more adaptable to compliance regimes that require providing reasons for decisions (for example, a law might grant consumers the right to an explanation of an automated decision). An adaptable system might include an internal logic trace or influence score for each decision, which can later be exposed via an interface if regulators or customers require it.
Continuous Monitoring and Model Management: Adaptive compliance means the AI system should evolve with its data and environment. Organizations should embed capabilities for continuous performance monitoring, bias detection, and drift detection in their AI systems (Shirkhanloo, 2025). If a model’s accuracy degrades or exhibits bias as input data changes over time, the system should flag this and allow for retraining or tuning. Designing an AI pipeline that supports regular updates (including the ability to roll back to a previous model if needed) will facilitate responding to new regulatory benchmarks. For example, if a future regulation sets a threshold on error rates for a medical AI device, a company that routinely monitors and retrains its model will be able to ensure it stays under that threshold. This kind of “living” AI system governance goes beyond static compliance checks – it requires automation and tools for real-time oversight. Dashboards that track key compliance metrics (like demographic parity in model outputs or latency in an AI vision system if safety standards mandate a response time) can alert teams to issues before they become violations. In essence, the system and its governance processes become self-correcting to some degree, which is crucial as regulatory goalposts shift.
Adaptive Governance Structures: On the organizational side, designing adaptability means having governance processes that can quickly integrate new requirements. Companies should establish cross-functional teams or committees that regularly review regulatory updates and assess what changes in AI systems or policies are needed (Shirkhanloo, 2025). For instance, if a new AI transparency law is enacted, this team should determine what additional information the AI needs to provide to users and work with engineers to implement it. Agile governance frameworks – where policies are revisited frequently rather than annually – ensure compliance keeps pace with external changes. Some firms are even creating “AI ethics and compliance boards” that include external experts to challenge and improve the firm’s AI practices continuously. Organizations can adapt with less friction by treating compliance as an iterative process (much like agile software development).
Vendor and Third-Party Adaptability: Many businesses rely on third-party AI services (for cloud AI, APIs, pre-trained models, etc.). Ensuring your overall AI ecosystem is adaptable means holding vendors accountable for compliance updates. As mentioned, contracts should require vendors to disclose changes. Additionally, companies might favor AI products that offer strong compliance support – for example, AI platforms that allow full export of decision logs or offer configurable bias mitigation tools will make it easier to adjust to new legal demands. If a particular vendor’s model becomes non-compliant due to a regulatory change, an adaptable strategy could involve having contingency plans (perhaps an alternate vendor or an in-house solution ready). Organizations should monitor the regulatory compliance of their supply chain, not just their systems (Shirkhanloo, 2025).
Building for the Future: A forward-looking design stance anticipates where regulation is heading. While not every detail can be predicted, specific trends are clear. For example, we expect more significant requirements around AI transparency, user consent, fairness, accountability, and security. Designing AI systems with robust user consent flows, explanation capabilities, bias audits, and security safeguards means you are likely already compliant when those aspects become formally regulated. Following ethical AI guidelines from bodies like the OECD or ISO technical standards (like the upcoming ISO/IEC 42001 for AI management) can be helpful as these often foreshadow regulatory expectations. By aligning system design with these high-level principles, a company ensures its AI is built on a solid ethical foundation that laws will likely reinforce.
Finally, it is worth noting that adaptability itself is an asset. In a rapidly changing environment, companies that can nimbly adjust their AI products will have an edge over those that must halt operations to re-engineer for compliance. Investing in adaptive systems now can prevent costly re-development later. Moreover, demonstrating to regulators that your AI governance is dynamic and responsive can build trust and goodwill. Regulators have indicated they want to see a commitment to ongoing oversight – for example, the EU AI Act explicitly mandates post-market monitoring and updates to risk management over a system’s life (ISACA, 2024). Designing your AI processes to include continuous improvement loops will default fulfill these kinds of obligations. In conclusion, by integrating flexibility, monitoring, and ethical design principles, businesses can create AI systems that remain compliant with today’s rules and can be efficiently realigned with tomorrow’s rules. This adaptability minimizes compliance risk and promotes long-term innovation because the organization can respond confidently to new regulatory challenges without stifling its AI initiatives.
Conclusion
AI regulation is quickly moving from theoretical to operational, and businesses must be prepared to navigate this new terrain. As we have discussed, compliance roadmaps for AI should include staying informed on major laws like the EU AI Act, establishing rigorous internal programs for AI governance, keeping detailed documentation, performing regular readiness checks, and designing AI systems with future requirements in mind. While requiring investment, these efforts ultimately enable more sustainable and trustworthy AI adoption. By aligning compliance with ethical AI practices, organizations avoid penalties and enhance the quality and fairness of their AI outcomes, building trust with customers and stakeholders. Substantial AI compliance and governance can become a competitive advantage: Companies that proactively meet high standards may gain easier access to global markets and earn consumer confidence in an era of rising scrutiny (Shirkhanloo, 2025).
Preparing for AI regulation is not a one-time project but an ongoing journey. The regulatory landscape will evolve as technology advances and society’s expectations of AI crystallize. Businesses of all sizes, from startups to multinationals, should view the compliance roadmap as an integral part of their AI strategy. Those who implement adaptive, responsible AI practices now will be well-placed to thrive amid whatever regulatory changes come next. In summary, the path forward involves a commitment to responsible AI innovation – developing AI with attention to legal requirements, ethical principles, and the flexibility to adjust when new rules arrive. By doing so, companies can harness AI’s benefits while respecting societal values and the rule of law, ensuring their AI journey is impactful and compliant.
Sources/Resources
- Babin, R. (2024, November 21). Global AI regulations: Beyond the U.S. and Europe. CIO. https://www.cio.com/article/3608168/global-ai-regulations-beyond-the-u-s-and-europe.html
- ISACA. (2024). Understanding the EU AI Act (White Paper). Information Systems Audit and Control Association. https://www.isaca.org/resources/white-papers/2024/understanding-the-eu-ai-act
- Kompella, K. (2024, July 11). Is your business ready for the EU AI Act? TechTarget. https://www.techtarget.com/searchenterpriseai/tip/Is-your-business-ready-for-the-EU-AI-Act
- Levi, S. D., Kumayama, K. D., Ridgway, W. E., Ghaemmaghami, M., & Neal, M. M. (2024, June 24). Colorado’s Landmark AI Act: What Companies Need To Know. Skadden, Arps, Slate, Meagher & Flom LLP. https://www.skadden.com/insights/publications/2024/06/colorados-landmark-ai-act
- Hilliard, A. (2024, August 21). Assessing the Impact of Algorithmic Systems. Holistic AI. https://www.holisticai.com/blog/assessing-the-impact-of-algorithmic-systems
- Shirkhanloo, A. (2025, February 19). Beyond compliance: The case for adaptive AI governance. IAPP (International Association of Privacy Professionals). https://iapp.org/news/a/beyond-compliance-the-case-for-adaptive-ai-governance/
- White & Case LLP. (2025, March 31). AI Watch: Global regulatory tracker – United States. White & Case. https://www.whitecase.com/insight-our-thinking/ai-watch-global-regulatory-tracker-united-states
© 2025 SSR Research and Development. This article is protected by copyright. Proper citation according to APA style guidelines is required for academic and research purposes.