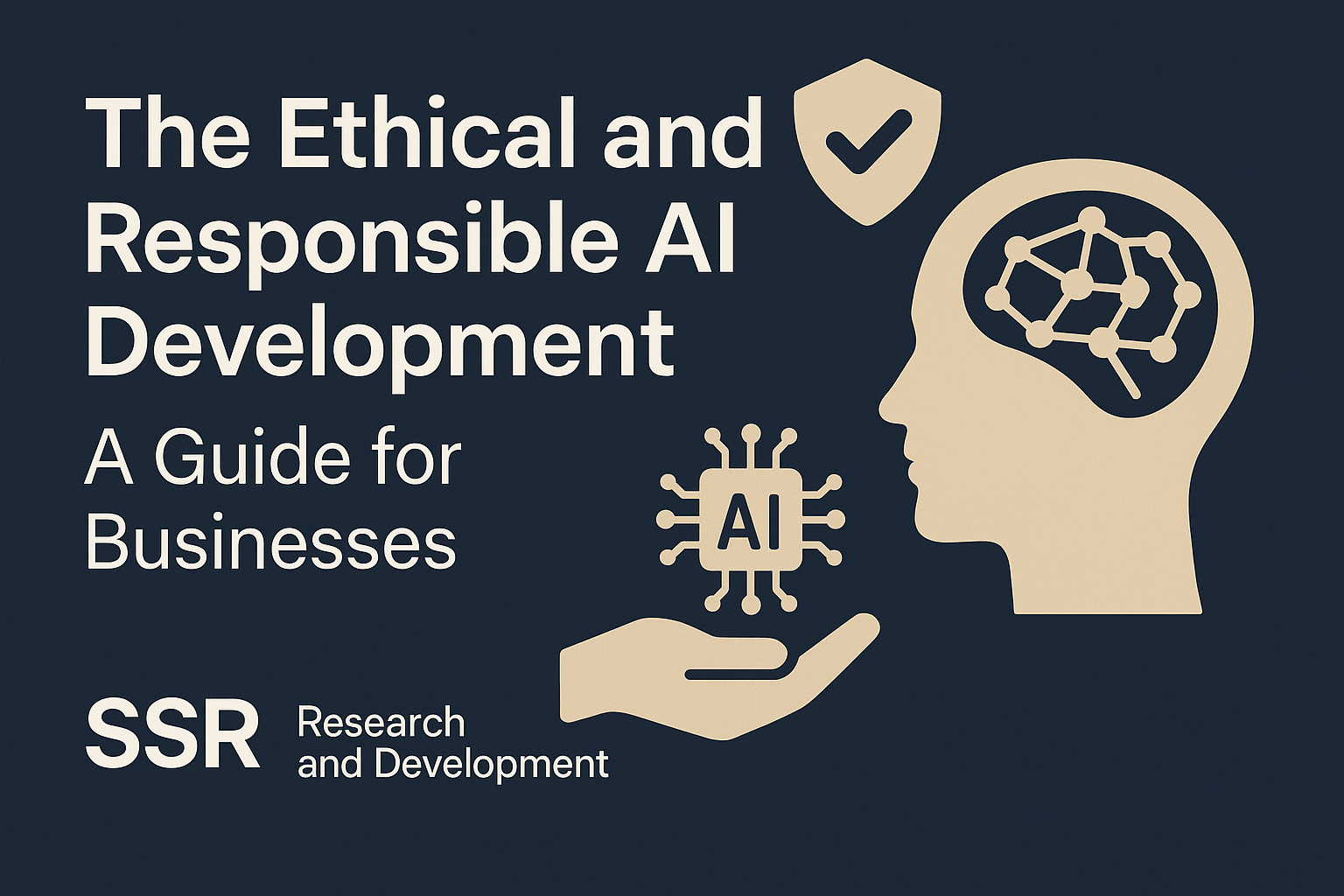
You can click on the Spotify podcast for a TL/DR. The sources generated for this post were done using Gemini 2.5 pro. The podcast was produced by NotebookLM.
Introduction: Artificial Intelligence offers transformative business opportunities across all industries. However, its adoption raises critical ethical considerations. Organizations of every size must ensure that AI systems are developed and deployed responsibly to maintain trust and avoid unintended harm. Public concern is high – over half of people believe AI companies fail to consider ethics during development. By proactively addressing ethical risks, businesses can enhance trust, comply with emerging regulations, and sustainably unlock AI’s benefits. This white paper provides comprehensive best practices and frameworks to guide ethical AI adoption, covering everything from design principles to governance structures. It emphasizes key values like transparency, accountability, fairness, privacy, and bias mitigation as foundations for responsible AI.
Why Ethical AI Matters for Businesses
Ethical AI is not just a compliance checkbox but fundamental to long-term business success. If AI systems behave unfairly or opaquely, organizations risk reputational damage, legal liability, and loss of customer trust. Conversely, responsible AI use can drive innovation and customer loyalty by aligning technology with human values. Key reasons for ethical AI matters include:
- Trust and Reputation: Businesses depend on user and stakeholder trust. Ethical lapses (e.g., biased hiring algorithms or privacy violations) can erode public confidence. Adopting transparent and fair AI practices builds a reputation for accountability and integrity.
- Risk Management: AI introduces novel risks – from biased decision outcomes to security vulnerabilities. Identifying and mitigating these risks early (through ethics guidelines, testing, and oversight) helps prevent costly failures. Frameworks like NIST’s AI Risk Management Framework treat AI ethics as part of overall risk management.
- Regulatory Compliance: Policymakers worldwide are enacting AI regulations and standards. For example, the EU’s upcoming AI Act and the U.S. NIST AI Risk Management Framework provide guidance for trustworthy AI. Companies implementing ethical AI practices now will be better prepared to meet future legal requirements and industry standards.
- Inclusive Innovation: Ethical AI ensures technology works for all groups in society, not just a few. By addressing biases and accessibility, businesses can reach underserved markets and avoid amplifying social inequalities. This leads to more inclusive products and broader adoption.
- Long-Term Sustainability: AI systems aligned with core values (like human rights, fairness, and safety) are more likely to deliver sustainable benefits. Ethical principles act as guardrails, ensuring AI serves business goals and societal well-being in the long run.
In short, prioritizing ethics in AI development is both a business necessity and a social responsibility. The following sections outline how to implement this through concrete best practices, awareness of common pitfalls, adherence to leading frameworks, and strong internal governance.
Best Practices for Ethical AI Design, Development, and Deployment
Implementing AI ethically requires attention at every stage of the AI lifecycle – from initial design through development and deployment. Below are best practices that businesses (small or large, in any industry) should follow to embed ethical principles into their AI systems:
- Establish Ethical AI Principles from the Start: At the design phase, clearly define a set of AI ethics guidelines aligned with your company’s values and relevant frameworks (for example, committing to fairness, transparency, privacy, and human-centric design). These principles will guide all project decisions and establish that ethical considerations are the top priority.
- Conduct Impact Assessments: Conduct an ethical impact assessment or risk analysis before development. Consider the AI system’s potential positive and negative impacts on individuals, communities, and society (e.g., could the system inadvertently discriminate or affect privacy?). Document these risks and plan how to mitigate them. Many frameworks call for analyzing context and societal impact as a first step (“Map” stage of NIST’s AI RMF).
- Diverse and Inclusive Design: Involve a diverse team of stakeholders in designing and reviewing AI systems. Different perspectives help spot ethical issues that homogeneous teams might miss. Inclusive design also means considering users of varied backgrounds and abilities – ensuring the AI will serve all intended users equitably.
- Privacy by Design: Embed privacy considerations into the system architecture. Limit data collection to what is necessary, use techniques like anonymization or encryption, and comply with data protection regulations. Communicate data usage policies to users or employees. Privacy-by-design reduces the risk of privacy violations or unauthorized data use.
- Data Governance and Quality: High-quality, representative data is critical to avoid bias. Establish robust data governance: vet datasets for biases or gaps, document data provenance, and ensure data is secure. If using personal data, obtain proper consent and follow regulations. Good data practices will directly impact the fairness and accuracy of AI outcomes.
- Mitigate Bias and Ensure Fairness: Rigorously test the AI model for algorithmic bias and unfair outcomes during development. Bias can occur if the training data reflects historical prejudices, leading to discriminatory decisions.Use fairness metrics and audits to detect disparate impacts on different groups. If biases are found, adjust the data or model (e.g., re-sample data, tweak algorithms) and continually re-test. Domain experts or ethicists should also be involved in reviewing decisions for fairness.
- Transparency and Explainability: Strive to make AI systems as transparent as possible—document how the model works, what data it uses, and what factors influence its outputs. Explain AI decisions through explainable AI techniques or simple rule-based descriptions, especially in high-stakes applications. Transparency builds user trust and makes debugging or auditing AI behavior easier.
- Human-in-the-Loop and Oversight: Design AI solutions with appropriate human oversight. Identify areas where human judgment should be retained or where an AI’s suggestion should require human approval (for instance, in hiring or medical decisions). Human-in-the-loop approaches ensure that AI remains a tool to augment human decision-making, not replace accountability. Clear escalation paths for exceptions or appeals (e.g., a customer can request a human review of an AI-made decision) are essential.
- Robustness and Safety Testing: Test AI systems for robustness and security before deployment. Assess how the system handles edge cases or adversarial inputs and ensure it behaves safely under different conditions. For AI controlling physical equipment or critical processes, include extensive safety tests. Also, resilience to cyberattacks (like adversarial attacks on ML models) should be evaluated. A robust AI is less likely to cause unintended harm or be exploited by bad actors.
- Gradual Rollout and Monitoring: When deploying AI, start with a pilot or phased rollout. Continuously monitor the AI’s outputs in real-world conditions. Set up metrics and feedback channels to catch bias, errors, or concept drift over time. Monitoring allows you to make iterative improvements and address problems early. If the AI makes a harmful mistake, have an incident response plan to investigate and remediate it.
- User Training and Change Management: Introduce AI tools alongside training for employees or users on their proper and ethical use. Educate staff about the AI’s capabilities and limitations and train them to recognize and flag potential issues (e.g., if they suspect the AI is biased or malfunctioning). Cultivating an informed workforce helps ensure AI is used responsibly and that humans remain engaged supervisors of AI behavior.
- Documentation and Transparency to Stakeholders: Maintain clear documentation for each AI system – including its purpose, design process, training data characteristics, performance benchmarks, and limitations. Consider publishing summaries of this information (model cards, datasheets, or transparency reports) to external stakeholders or customers as appropriate. Being transparent about your AI practices demonstrates accountability.
Continuous Improvement and Governance: Ethical AI is an ongoing commitment. Regularly review AI systems against your ethical principles and new developments (e.g., updated regulations or standards). Schedule periodic audits for compliance with fairness, privacy, and security criteria. Incorporate user feedback and keep refining the system and your processes. This ties into a strong governance structure, which is covered later in this paper. By following these best practices, organizations can integrate ethical checks and balances throughout the AI development lifecycle. This proactive approach dramatically reduces the likelihood of ethical lapses and builds a culture of responsibility around AI innovation.
Common Ethical Challenges in AI and How to Mitigate Them
Even with best practices, particular ethical challenges are common when adopting AI. Awareness of these issues is the first step; implementing targeted mitigation strategies is the next. Below, we identify key ethical challenges businesses face with AI and ways to address each:
- Bias and Discrimination: AI systems can inadvertently perpetuate or amplify biases, leading to unfair or discriminatory outcomes. For example, a hiring AI might favor males if trained on biased past data, or a loan algorithm might disfavor specific zip codes. Mitigation: To tackle bias, use diverse and representative training data and audit algorithms for disparate impacts.Regularly test outcomes across demographic groups. If you find skewed results, adjust the model or apply fairness constraints. Involve ethicists or affected groups in reviewing decisions. A culture of inclusivity (in both the development team and the AI’s design) helps ensure fairness.Ultimately, bias mitigation is an ongoing process: Continuously monitor and update the AI to improve its fairness over time.
- Lack of Transparency (“Black Box” Effect): Many AI models (incredibly complex machine learning like deep neural networks) operate as “black boxes” that even developers struggle to interpret. This opacity can erode trust – users and stakeholders may not understand how or why a decision was made, which is problematic in sensitive applications (finance, healthcare, etc.). Mitigation: Strive for explainable AI. Use algorithms and tools to provide interpretable outputs (e.g., decision trees and model-agnostic explainers like SHAP/LIME for complex models). Provide clear explanations or reasons for decisions in user-friendly terms whenever possible. Documentation is key: maintain records of model logic, feature importance, and known limitations. Also, set expectations with users about when an AI is involved in decisions. Transparency and explainability are core principles in major AI ethics frameworks and are crucial for accountability.
- Privacy and Data Protection: AI often relies on large datasets, some containing personal or sensitive information. This raises concerns around privacy – misuse of personal data, surveillance, or unauthorized sharing. There is also the risk of violating data protection laws if AI handles personal data inappropriately. Mitigation: Adopt privacy-by-design principles. Minimize the collection of personal data and avoid sensitive attributes if not needed for the AI’s task. Where personal data is used, it must be secured (encryption, access controls) and ensure compliance with regulations (GDPR, HIPAA, etc.). Implement data anonymization or federated learning to reduce exposure to individual data. Communicate to users what data is collected and why. Internally, enforce data handling policies and train employees on data ethics. By building robust data privacy and security measures, organizations protect users and reduce the chance of harmful data leaks or abuses.
- Security and Malicious Use: AI systems can be targets of attack or misuse. Adversaries might tamper with an AI (e.g., poison the training data or input adversarial examples to fool the model), potentially causing harmful outcomes. Additionally, malicious actors can amplify cybersecurity threats using AI tools (like deepfakes or automated hacking). Mitigation: Treat AI security as part of your overall cybersecurity program. Protect training and input data integrity – for example, validate and sanitize inputs and monitor for anomalous behavior that could indicate manipulation. Use adversarial training techniques for critical models to improve their resilience against attacks. Keep AI software and libraries updated to patch vulnerabilities. Limit authorized users’ access to AI models and APIs to prevent abuse. Also, create a scenario plan for potential misuse of your AI (could someone repurpose it for harm?) and put safeguards or usage policies in place to prevent that. Robust testing and cybersecurity measures are essential to ensure AI systems cannot be easily compromised. Failing to secure AI can lead to financial losses and reputational damage. So, this risk must be managed proactively.
- Accountability and Legal Liability: When AI systems make decisions that significantly affect people, a key question arises: Who is accountable for the outcomes? The organization must take responsibility if an automated decision causes harm (e.g., an unsafe autonomous vehicle or a wrong financial decision). There is a risk that humans defer too much to AI (“automation bias”) and do not exercise proper oversight, leading to unchecked errors. Mitigation: Establish clear accountability frameworks for AI decisions. Even if AI makes automated choices, assign a responsible human owner for each AI system or decision domain. Define escalation procedures for exceptions. Documentation and transparency help with accountability – assigning responsibility and correcting issues is more manageable if you can explain how a decision was made. Some organizations create AI ethics review boards or designate an AI ethics officer to oversee high-stakes use cases. The principle of accountability is enshrined in frameworks like the OECD AI Principlesand ISO AI governance standards, which urge that AI decisions be traceable and auditable. In practice, accountability means blaming the AI or vendor and ensuring your company has governance to answer for and rectify any AI-driven harm.
- Misuse and Unintended Consequences: AI systems can be used in ways the designers did not intend. For instance, a facial recognition tool might be deployed for mass surveillance violating civil liberties, or a chatbot could be manipulated to generate harmful content. Even well-intended AI can have unintended societal effects (e.g., affecting employment patterns or influencing public opinion). Mitigation: Anticipate potential misuses during the design phase (threat modeling for ethics). Include usage restrictions in your AI system’s design or terms of service (for example, some companies watermark AI outputs to detect deepfakes). Monitor how your AI is used in the field and be ready to intervene if it is being misused. Engage with external stakeholders – ethicists, user communities, and regulators – to get feedback on unanticipated consequences. By actively monitoring and governing the use of AI, organizations can catch and prevent misuse before it causes serious harm.
Note: Many of these challenges are interrelated. For example, lack of transparency can exacerbate bias (since biased results might go unnoticed), and poor security can lead to privacy breaches. Therefore, an integrated approach is needed—addressing ethics holistically rather than treating each issue in isolation. The following section introduces established frameworks and standards that provide structured approaches to managing these ethical risks comprehensively.
Leading Frameworks and Standards for Ethical AI
Multiple international frameworks and standards have been developed to help organizations implement AI ethically and responsibly. These frameworks encapsulate principles, best practices, and governance processes that are widely recognized. Businesses can look to these resources for guidance and even benchmarking their AI ethics programs. Below, we briefly describe some of the leading frameworks and standards:
OECD AI Principles (OECD Recommendation on AI)
One foundational set of guidelines is the OECD AI Principles, introduced by the Organisation for Economic Cooperation and Development in 2019 and affirmed by 47 countries (including G20 nations). These principles (the first intergovernmental AI standard) promote the development of “trustworthy AI that respects human rights and democratic values.”. The OECD Principles outline five core value-based requirements for AI: inclusive growth, sustainable development, and well-being; human-centered values and fairness (respect for human rights, diversity, and privacy); transparency and explainability; robustness, security, and safety; and accountability. These succinctly capture the ethical priorities for AI – from ensuring AI benefits society and does not discriminate to making AI systems transparent, secure, and answerable for their actions. The OECD also guides policymakers (e.g., investing in R&D, fostering an AI ecosystem, and international cooperation), which complements the value principles. The OECD Principles serve as a high-level framework of ethical AI goals for businesses. Companies can map their internal AI policies to these principles to ensure they align with global norms. Notably, the OECD definition of an AI system and its lifecycle is widely used and has informed regulations like the EU AI Act. The OECD continues to update these guidelines (most recently in 2024) to address new developments in AI.
NIST AI Risk Management Framework (AI RMF 1.0)
The U.S. National Institute of Standards and Technology (NIST) released the AI Risk Management Framework 1.0 in January 2023. While voluntary, this framework provides a practical, detailed approach to evaluating and managing AI risks. It is built on the premise of “trustworthy AI.” It identifies seven characteristics of trustworthy AI: safe, secure and resilient, explainable and interpretable, privacy-enhanced, fair (with bias managed), accountable and transparent, and valid and reliable. These qualities closely mirror the ethical principles discussed earlier, translating them into attributes that AI systems should meet.
- Map: Identify AI contexts, stakeholders, and risks (e.g., what could go wrong and who might be affected).
- Measure: Analyze and assess identified risks using metrics and tools to quantify fairness, performance, security, etc.
- Manage: Prioritize risks and take actions to mitigate them (through controls, system improvements, etc.), then track ongoing effectiveness.
- Govern: Oversee the AI risk management program, establishing policies, roles, processes, and a culture that continuously supports the Map-Measure-Manage functions.
Governance is considered the cornerstone (deliberately placed at the center of the NIST RMF diagram), emphasizing that technical risk management will fall short without organizational governance and accountability. The NIST framework is comprehensive and aligns well with other frameworks like the OECD and upcoming regulations. For businesses, adopting NIST’s AI RMF can provide a step-by-step process to implement ethical AI, ensuring that considerations such as fairness, privacy, and security are systematically evaluated at each phase of AI adoption. NIST also offers an online AI RMF Playbook with actionable guidance for each sub-function and a crosswalk mapping the framework to other standards. Using the NIST RMF, even on a smaller scale, can significantly improve an organization’s ability to identify ethical risks and address them proactively as part of enterprise risk management.
ISO/IEC AI Standards (SC 42)
The International Organization for Standardization (ISO) and the International Electrotechnical Commission (IEC) have a joint committee (JTC 1/SC 42) dedicated to AI standards. This group is developing international standards for AI governance, risk management, and technical robustness. Two notable standards are:
- ISO/IEC 42001 (AI Management System Standard): Published in 2023, ISO 42001 specifies requirements for an AI Management System (AIMS) within an organization. Like how ISO 9001 guides quality management, ISO 42001 provides a framework for establishing, implementing, and continually improving a management system specifically for AI. Key components of ISO 42001 include requirements for ethical risk mitigation, bias management, data quality, security, training, and ongoing monitoring. Implementing ISO 42001 can help a business ensure it has the proper governance and processes to deploy AI responsibly. The benefits of adopting ISO 42001 include enhanced trust and transparency by building accountability and ethical considerations and improved risk management through structured procedures. Organizations may seek certification to ISO 42001 to demonstrate that their AI practices meet this global standard.
- ISO/IEC 38507 (Governance of AI): Published in 2022, ISO 38507 provides guidance for organizations’ governing bodies on the oversight of AI. It addresses the governance implications of using AI, ensuring that boards and executives align AI initiatives with organizational values and objectives. The standard emphasizes “promoting accountability, transparency, and ethical considerations” in AI use. In practice, ISO 38507 suggests that leadership should treat AI as a part of corporate governance – setting clear responsibilities for AI outcomes, aligning AI projects with business strategy, managing risks (ethical, technical, and operational), and ensuring proper controls. Following ISO 38507, companies can strengthen their internal governance structures to enable effective and acceptable AI use. It complements ISO 42001 by focusing on top-level oversight and policy, whereas 42001 focuses on operational management systems.
In addition to these, SC 42 has produced standards on AI vocabulary and concepts (ISO 22989), an AI lifecycle framework (ISO 23053), and various technical reports on issues like bias in AI (ISO/IEC TR 24027) and AI robustness testing (ISO/IEC 24029). ISO/IEC AI standards collectively aim to provide a comprehensive toolkit so organizations can standardize their approach to AI ethics and risk worldwide. Businesses should keep an eye on these standards – even if not formally adopting them, they offer valuable best practices and may inform future regulatory expectations.
Other Notable Frameworks and Initiatives
Beyond OECD, NIST, and ISO, other frameworks can guide ethical AI development:
- EU Ethics Guidelines for Trustworthy AI: In 2019, the European Union’s High-Level Expert Group on AI defined seven key requirements for trustworthy AI (including human agency, technical robustness, privacy, transparency, non-discrimination, societal well-being, and accountability). Though not law, these guidelines influenced many corporate AI ethics charters and fed into the EU’s risk-based AI Act regulation.
- U.S. Blueprint for an AI Bill of Rights: Released by the White House in 2022, this is a set of principles to protect the public in the AI era (focused on safe and effective systems, freedom from bias, data privacy, notice explanation, and human alternatives). It provides a user-centric lens that businesses can consider, especially for consumer-facing AI.
- IEEE Ethically Aligned Design & IEEE 7000 Series: The IEEE has published extensive work on ethically aligned AI design and specific standards (IEEE 7001-7008 series) addressing issues like algorithmic bias, transparency of autonomous systems, and data privacy processes. These can be resources for technical teams looking for engineering-level guidance on implementing ethics in AI systems.
- Industry Principles: Many companies have created AI ethics principles (Google’s AI Principles, Microsoft’s Responsible AI Standards, etc.). While these are internal, they often mirror the above frameworks and can provide practical examples. Cross-industry collaborations like the Partnership on AI also release research and best practices (for instance, on algorithmic fairness or AI explainability techniques).
Synthesis: The encouraging news is that a broad consensus is emerging across these frameworks. There is alignment on what responsible AI entails – values like transparency, fairness, privacy, safety, and accountability are universally emphasized. The frameworks differ in focus (some are high-level principles, some are detailed processes), so businesses may choose the most suited to their needs. For example, a small startup might use the OECD principles as a moral compass. In contrast, a large enterprise or regulated industry firm might adopt the NIST or ISO approaches for rigorous risk management and auditability.
In many cases, organizations will use a combination of these resources. The key is to internalize the spirit of these frameworks, ensuring that AI ethics are systematically woven into how AI is built and used. The following section discusses creating internal governance structures to do precisely that.
Building an Internal AI Governance Structure
To operationalize ethical AI, companies should establish internal governance structures and processes that oversee AI initiatives. Governance provides the organizational framework to enforce the principles and practices discussed. Here are recommendations for creating robust AI governance within an organization:
- Define and Adopt AI Ethics Principles: Start by formalizing a set of AI ethics principles for your organization (often published as an “AI Ethics Charter” or guidelines document). This should be endorsed by leadership and communicated to all employees. The principles can be based on the frameworks above (e.g., commit to fairness, transparency, privacy, accountability, etc.) and tailored to your company’s mission. A clear set of declared principles sets the expectation that every AI project must uphold these values.
- Establish an AI Ethics Committee or Review Board: Create a cross-functional team responsible for AI ethics governance. This AI Ethics Committee (or board) should include senior executives, technical leads, legal/compliance, and representatives from diverse backgrounds (to bring varied perspectives). The committee’s role is to review high-risk AI projects, monitor compliance with AI principles, and provide guidance on ethical dilemmas. For example, before deploying an AI system to make hiring decisions, the committee should assess its fairness and approve its use. This review process injects oversight and accountability at key decision points.
- Integrate Ethics into AI Project Lifecycle: Require that every AI project goes through ethics checkpoints. This could involve an initial ethics risk assessment during design (as discussed earlier), periodic check-ins with the governance team during development, and final approval before deployment. Create simple tools or checklists for teams to evaluate ethical considerations (for instance, a questionnaire about potential biases, impacts, privacy issues, etc.). Embedding these steps into the standard project workflow ensures ethics are considered alongside performance and business objectives, not as an afterthought.
- Appoint Responsible AI Roles: Consider designating specific roles or champions for AI ethics. Some organizations have a Chief AI Ethics Officer or add these duties to a Chief Data Officer’s role. Even without a C-suite title, you can assign “Responsible AI Leads” within business units – people with AI ethics training who can liaise with the central committee. The goal is to have identified owners for AI ethics efforts so that it is someone’s job (and performance metric) to uphold ethical standards. These leaders also signal top-down commitment.
- Employee Training and Awareness: Governance is ineffective if the people developing and using AI are unaware of ethical practices. Regularly train employees (especially engineers, data scientists, and product managers) on responsible AI development. This can include workshops on topics like bias in AI, privacy-preserving techniques, or relevant regulations. Also, share case studies of AI ethics failures and successes to illustrate their importance. An ethically aware workforce will be better equipped to spot issues early and champion the company’s principles. Additionally, encourage an open culture where employees can voice concerns about AI projects without fear – maybe even implement an ethics issue reporting channel.
- Documentation and Model Tracking: Good governance requires documentation. Maintain an inventory of all AI models in use, along with documentation of their purpose, training data, validation results, and any ethical risk assessments done. As recommended by the NIST “Govern” function, this inventory approach ensures the organization has visibility into where AI is used and how. It also helps in auditing and responding to incidents. Version control and change logs for models are also important – if a model is updated, note what changed and if any new ethical review was conducted.
- Continuous Monitoring and Audit: Governance does not end at deployment. Set up processes to monitor AI systems in production for compliance with ethical standards. For instance, periodically test a live model for bias drift (has it become less fair over time?), check that privacy controls remain effective, and verify that outputs stay within acceptable bounds. Some companies establish internal audit teams or bring in third-party auditors to evaluate their AI against criteria (much like financial audits). Regular audits can verify that the reality of AI use matches the intended ethical design. If audits or monitoring find issues, have a procedure to escalate and remediate – possibly involving pausing or retraining the system.
- Ethical Incident Response: Despite best efforts, things can go wrong. An AI system might behave unexpectedly or cause an incident (e.g., a public relations issue due to an offensive output). Prepare an incident response plan for AI-related issues, similar to a cybersecurity incident plan. This should define how to investigate the problem, who needs to be informed (up to executive level), how to communicate externally if needed, and how to prevent recurrence. Transparency is part of accountability – if a significant incident occurs, being honest about it and fixing it will maintain more trust than trying to cover it up.
- Align AI Governance with Corporate Governance: Integrate AI ethics into corporate governance and risk management structures. For example, AI risks should be included in enterprise risk registers, the Board of Directors should be briefed on AI ethics progress and risks, and internal audit or compliance teams should have AI expertise. The tone from the top matters: When executives ask about and reward ethical AI practice, it becomes a priority throughout the organization. Governance frameworks like ISO underline that AI is now a boardroom issue.
- Stay Informed and Adapt: The AI landscape and regulatory environment are evolving rapidly. A governance structure should continuously update its policies in light of new laws (such as the EU AI Act or sector-specific rules), emerging standards, and lessons from others’ experiences. It is wise to participate in industry groups or forums on AI ethics to share knowledge. Some companies form external advisory councils, bringing academic or civil society experts to get outside perspectives on their AI plans. Your governance approach will remain effective as technology and norms change by staying proactive and adaptable.
In summary, internal governance operationalizes ethical principles through concrete organizational mechanisms. It creates a system of checks and balances around AI development, much like financial governance does for accounting. With strong governance, businesses can confidently pursue AI innovation while managing ethical risks and upholding public trust.
Key Ethical Principles in Practice: Transparency, Accountability, Fairness, Privacy, and Bias Mitigation
Throughout this paper, we have highlighted several fundamental principles that underpin responsible AI. Here, we recap and emphasize the importance of five key principles – Transparency, Accountability, Fairness, Privacy, and Bias Mitigation – and discuss how businesses can uphold each in practice:
- Transparency: Transparency means being open about how AI systems work and how decisions are made. It is crucial for building trust – users and stakeholders are more likely to accept AI outcomes if they understand the reasoning behind them. In practice, transparency involves providing information and explanations. For instance, if an AI model recommends denying a loan, the user should be informed that an algorithm made that decision and given an explanation (e.g., “application lacked sufficient credit history”). Internally, developers should document model design and decision logic. Transparency also extends to communicating where and why AI is used in the business. Leading frameworks treat transparency and its technical counterpart explainability as core requirements. By prioritizing transparency, businesses make their AI less of a “black box,” which helps identify errors, facilitates accountability, and empowers users. It is essential in sensitive applications to provide explanations that are understandable to the affected individuals.
- Accountability: Accountability ensures that some identifiable persons or processes take responsibility for AI outcomes. AI should not be an excuse to shrug off blame (“the algorithm did it”). This principle is vital for ethical governance – it underpins the idea that humans remain in control of and responsible for AI. Organizations should establish clear ownership for each AI system (who oversees it) and decision (who approves it) to implement accountability. Mechanisms like audit logs and traceability of AI decisions support accountability by showing what the AI did and why. An accountable organization will investigate and address the issue if something goes wrong rather than obscuring it. Accountability also means compliance with applicable laws – for example, anti-discrimination laws still apply to AI-driven decisions, and the company will be held liable for violations. Both the OECD and ISO governance standards highlight accountability as a key principle. When businesses embed accountability, they create a culture where ethical AI is everyone’s responsibility, and answerability is up the chain for maintaining those standards.
- Fairness: Fairness in AI refers to the absence of unjust biases or discrimination in the system’s operations. An AI system is fair if it delivers equitable outcomes for different groups unless justified by relevant differences. Unfair AI can lead to excluded or negatively impacted populations (e.g., an AI consistently giving lower-paying job assignments to one demographic). Ensuring fairness is an ethical and often legal imperative (to comply with non-discrimination laws). Businesses should define fairness for each AI application (there are different technical definitions of fairness – e.g., equal prediction accuracy across groups, demographic parity in outcomes, etc., depending on context). Steps to ensure fairness include the bias mitigation practices we discussed: use representative data, avoid encoding sensitive attributes, test results for bias, and involve diverse perspectives in design. Fairness also involves procedural fairness – treating people with transparency and allowing recourse (for example, giving candidates rejected by an AI hiring filter a chance for human review). By operationalizing fairness, companies reduce the risk of discriminatory outcomes and help AI act as a force for inclusion rather than exclusion.
- Privacy: Privacy is a fundamental right and ethical concern whenever AI uses personal data. Respecting privacy means handling personal information with care, only using it in ways that individuals have consented to (or that have legitimate basis), and protecting it from misuse. For AI, privacy issues arise in data collection, analysis, and even inferring data (some AI can infer sensitive attributes). Businesses must implement strong data protection measures for AI projects: follow data protection principles like purpose limitation (use data only for the stated AI purpose), data minimization (do not hoard unnecessary personal data), and retention limits (delete data when no longer needed). Security measures (encryption, access control) are critical to prevent breaches. Also consider privacy-preserving machine learning techniques – for example, federated learning (where raw data stays on user devices) or differential privacy (adding noise to outputs to protect individual data). Being transparent to users about data use and giving them control (opt-outs, privacy settings) where feasible further demonstrates respect for privacy. Upholding privacy is ethical; it also keeps you compliant with laws and maintains user trust – people will quickly lose confidence in an AI system (or brand) if they feel their data is mishandled.
Bias Mitigation: Bias mitigation is closely tied to fairness but is worth stating as its ongoing principle. Because biases can sneak in at many stages (data collection, model selection, interpretation of results), organizations must be vigilant in identifying and reducing bias continuously. This principle acknowledges that no system is unbiased, but you can strive to detect and correct biases to the greatest extent possible. Mitigation strategies include those already noted: diverse training data, algorithmic fairness techniques, human review of results, and inclusive design. It is important to mitigate institutional bias – for example, if a company historically undervalued specific customer segments, that bias might reflect in AI unless consciously countered. Regular bias audits and updates are akin to performing “maintenance” on the ethical quality of the AI.
Furthermore, bias is not just about protected classes (like race or gender); it can also mean avoiding unfair biases based on geography, socio-economic status, or other ethically relevant factors. Effective bias mitigation improves the AI’s accuracy and fairness, ensuring it works well for various scenarios and people. It directly supports the principle of fairness and helps avoid ethical and legal pitfalls. As one expert succinctly put it, we must ensure that in a world driven by algorithms, “the algorithms are doing the right things… the legal things… and the ethical things.”
These principles are interdependent. For example, transparency enables accountability and bias mitigation furthers fairness. Privacy can sometimes conflict with transparency (you cannot be transparent about individual data decisions without risking privacy), so a balance must be struck. Businesses create AI systems worthy of users’ trust by institutionalizing these principles in policies and everyday practice. They serve as a north star when tough trade-offs arise – e.g., if a highly accurate model is not explainable, transparency principles may lead you to choose a slightly less complex model that can be explained. Alternatively, if a new customer analytics AI could maximize profit but intrude on privacy, the privacy principle might guide you in refining the approach. In essence, these ethical principles help ensure that the pursuit of AI innovation remains aligned with human values and societal norms.
Conclusion
AI technology is advancing rapidly, but without ethical and responsible development, its promise could be undermined by public mistrust, harm to vulnerable groups, or regulatory backlash. Businesses that proactively embrace ethical AI practices will be better positioned to innovate confidently, gaining a competitive edge by building AI systems that customers, employees, and regulators can trust. This white paper has outlined how companies of all sizes can operationalize ethical AI – from adopting best practices in design and development to recognizing common pitfalls and addressing them, leveraging global frameworks as guides, and establishing robust internal governance.
The journey to ethical AI is continuous. It requires commitment from the C-suite to the engineering teams and a willingness to adapt as we learn more about AI’s impacts. Nevertheless, the effort is well worth it. Responsible AI fosters accountability and excellence, pushing teams to consider quality multidimensionally (not just accuracy or efficiency, but fairness, transparency, etc.). It also opens opportunities – for example, having strong AI governance can become a selling point to clients or a requirement to participate in specific markets (where RFPs increasingly ask about AI ethics compliance).
In closing, by grounding AI initiatives in core principles like transparency, accountability, fairness, privacy, and bias mitigation, businesses can ensure that their use of AI remains human-centered and beneficial. Ethical AI is not a one-time checkbox but a journey of continuous improvement and alignment with societal values. With thoughtful application of the guidance and resources discussed, organizations can harness AI’s power responsibly and sustainably to benefit their enterprise and the communities they serve.
Resources and References
Below is a list of frameworks, standards, and reference materials cited in this paper for further reading and implementation:
- OECD AI Principles (2019, updated 2024): OECD Council Recommendation on Artificial Intelligence – An intergovernmental framework of 5 values-based principles for trustworthy AI and policy recommendations.
- NIST AI Risk Management Framework 1.0 (2023): A comprehensive risk management approach for AI with core functions “Map, Measure, Manage, Govern” and characteristics of trustworthy AI. (NIST AI RMF official page*).
- ISO/IEC 42001:2023 (AI Management System): International standard specifying requirements for managing AI within an organization (Artificial Intelligence Management System) – includes processes for ethical risk mitigation, bias, data quality, etc.
- ISO/IEC 38507:2022 (Governance of AI) guides organizational governing bodies to oversee AI use, align AI with objectives, and ensure accountability, transparency, and ethics in AI governance.
- EU Ethics Guidelines for Trustworthy AI (2019): EU High-Level Expert Group’s guidelines outline seven key requirements for ethical AI in practice. (Link: EU Trustworthy AI Guidelines*)
- White House Blueprint for an AI Bill of Rights (2022): U.S. framework of five principles to safeguard the public in AI deployments (safe and effective systems, algorithmic discrimination protections, data privacy, notice and explanation, human alternatives). (Link: AI Bill of Rights*)
- IEEE Ethically Aligned Design & 7000-Series Standards: IEEE’s comprehensive work on AI ethics and specific technical standards (e.g., IEEE 7001 for transparency). (Link: Ethically Aligned Design (IEEE)*)
- Harvard Business School – Ethical AI in Business: Article on ethical considerations (bias, transparency, privacy, etc.) in business AI use, with practical examples.
- Brookings – NIST AI RMF Commentary: Analysis of NIST’s AI Risk Management Framework and its significance.
- Markkula Center – Ethics in the Age of AI (2023 Survey): Research on public attitudes toward AI ethics (trust, regulation, etc.) indicating 55% believe companies do not consider ethics.
© 2025 SSR Research and Development. This article is protected by copyright. Proper citation according to APA style guidelines is required for academic and research purposes.